Podcast: Play in new window | Download
Subscribe: RSS
Episode 19: Proxy Variables – Show Notes
This quick, informational segment introduces the concept of proxy variables. In short, proxy variables are data elements used in place of something that may be more pertinent but also more difficult to measure. It also touches on confounding and lurking variables – in case you wanted a dose of statistics theory this week.
In the continuing effort to show that correlation does not mean causation, we go through an example of a confounding variable, which might otherwise be mistaken for a proxy variable.
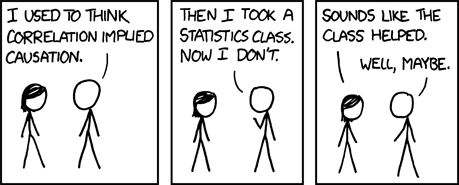
Also, whenever I think about confounding variables, this scene from Disney’s The Sword in the Stone comes to mind with Merlin screaming “Confound it!”

@DisneyRochelle’s weheartit
Additional Links for Proxy Variables
Proxy (statistics) Requisite Wikipedia link with the full definition
ACSH Explains ‘Confounding’: Why Correlation Does Not Mean Causation American Council on Science and Health has a great article on confounding variables and spurious correlations
Televisions, Physicians, and Life Expectancy 1994 paper by Rossman with the confounding variable example of TV, life expectancy, and physicians
A Fascinating Sign of High IQ PsyBlog article showing how emotional intelligence points to general intelligence – more needs for proxy variables
View episode transcriptWelcome to the Data Science Ethics Podcast. This is Lexy and today we’re talking about Proxy Variables. Proxy variables are data points that are used in place of other information that is either impossible or impractical to measure.
Let’s take an example of colleges trying to predict academic success as part of the admissions process. They may have hypotheses about some of the independent variables or factors that would indicate a higher chance of a good performance. For instance, they might consider prior academic performance by looking at high school transcripts and grade-point averages. They might think about fit with the school. Maybe they would look at the extracurricular activities of the student to assess how many of those activities are available on campus. They may want to ensure that the student has academic interests aligned with curricula. That could lead the admissions board to consider which major a student intends to pursue.
More generally, they may want to measure the student’s intelligence. However the concept of “intelligence” is a very difficult one to quantify. It is impossible to truly measure intelligence directly. Instead, they might use standardized test scores as a proxy variable for intelligence.
There are always usually some drawbacks to using a proxy variable rather than being able to measure the variable of interest directly. In our example with standardized test scores, one drawback might be that some people have difficulties taking tests. Their true intelligence would not be adequately reflected in the proxy variable of test scores. Data scientists have to carefully consider whether the information gained by including the proxy variable is sufficient given the drawbacks or if other information is necessary. In this instance, data scientists might look to include an indicator of test anxiety or some other factors that would influence test performance.
There is another dark side to proxy variables – and those are called confounding variables. Confounding variables are highly correlated to the one being predicted. But correlation does not mean causation. Usually these are outcomes from the same underlying cause – or lurking variable.
The example I always think of was one that my high school statistics teacher told us about. There was a study that came out showing a correlation between the number of televisions and the average life expectancy in nations around the world. Looking at just these two factors, it would seem like the more televisions a country had, the longer people lived. And while that was all true, it did not mean that bringing more televisions into the country would make people live longer.
In this case, the number of televisions would be a confounding variable to the life expectancy. The lurking variable, the root cause of both, is the wealth of the nations. Countries with more wealth tend to have better living conditions, sanitation, and access to medical care. They also tend to have the money for more televisions.
That’s not to say that there aren’t good uses for proxy variables. Many fields use proxy variables to great effect. For instance, think about the measurement of health. “Health” is another difficult concept to pin down. There are so many aspects to it. Yet the healthcare field uses all sorts of proxy variables like blood pressure, BMI, resting heart rate, any number of lab tests, and so forth. None of these is a direct measure of health. Instead, they are all proxy variables for aspects of it.
In our next episode, we’ll talk about a way that proxy variables are used in business every day to make decisions about the pricing of products and services.
Thanks for joining us for this episode of the Data Science Ethics Podcast. See you next time.